Harmonisation methods
There are different methods which can be used to harmonise data between studies. This section gives a brief introduction to some of the methods to make data more comparable.
The methods highlighted in this section mainly refer to retrospective harmonisation, where existing data is processed to be more comparable.
Manually choosing similar variables or individuals
This approach involves finding similar variables in the different datasets and cleaning them in a consistent way so that they can be compared.
This method might also involve reducing the sample in the studies to those individuals who would be more comparable on the variables of interest.
For example, this PLOS Medicine paper by Johnson, Li, Kuh and Hardy (2015) uses data from the CLOSER harmonised dataset on body composition excluded groups from their analytical sample who were likely to have particularly different body mass index (BMI) values.
This allowed comparative analyses and to see trends in body mass over time.
Equivalent scaling or categorisation
This approach involves putting the variables of interest on the same scale or using the same categories. This means that similar variables that were measured slightly differently are now comparable. A limitation of this method is that it can lead to less informative scales being used, as the scale or categories that are chosen are normally the lowest common denominator and so might not have the granularity of the more informative scales (Bann et al. 2022).
An example of equivalent categorisation for marital status is displayed below. Note how the final categorisation has to combine multiple categories from Study 1 to match Study 2 and how this affects the granularity of the data.
Study | Marital status | Marital status – equivalent categorisation |
---|---|---|
Study 1 | 1 = Single 2 = Single with a partner 3 = Married 4 = Divorced 5 = Separated 6 = Widowed |
1 = Single / Single with a partner 2 = Married 3 = Divorced / Separated 4 = Widowed |
Study 2 | 1 = Single 2 = Married 3 = Divorced or separated 4 = Widowed |
There are different ways in which equivalent categories or scaling can be achieved. You can explore these below:
Identify similar variables with Natural Language Processing
Natural language processing (NLP) is a branch of computer science and linguistics. It describes the process of computers understanding and analysing text and spoken words, which is enabled through machine learning and artificial intelligence.
NLP has been employed by the Harmony project to harmonise mental health-related questionnaires. The NLP in Harmony is applied to questionnaire items (questions) and determines which items are more semantically similar and which are more different, matches similar items, and assigns the match a score.
Use latent variable approaches to confirm two variables are similar
There are multiple statistical techniques which involve modelling a selection of items or variables as representing the same underlying construct.
For example, a questionnaire to assess depression has multiple questions asking about different feelings and behaviours that are often connected to being depressed. Answers to these questions gives us an idea of the underlying depression construct, even though we can’t see or measure depression itself.
Some of these statistical methods include:
- Structural equation modelling
- Factor analysis e.g. confirmatory factor analysis, moderated nonlinear factor analysis
- Item response theory
These latent variable modelling techniques normally allow for the testing of measurement invariance – a formal statistical test for the equivalence of measurement between the different items or variables.
Read more about measurement invariance in the Methodological guidance section.
Use a statistical algorithm to convert one variable into another
There are a selection of statistical methods used to harmonise data by converting scores on one scale into equivalent scores on another scale. They use a particular algorithm or set of rules to carry out this transformation. In this way there are similar to manual equivalent scaling or categorisation but use statistical rules to decide which values or categories are equivalent.
For example, if an individual scores 10 on one scale of depression (e.g. the Malaise-24), the algorithm determines that the equivalent score on the other scale (e.g. the GHQ-12) is 15.
These techniques are useful when different measurement tools have been used in different studies but are measuring the same underlying construct.
Some of these statistical methods include:
- Equipercentile linking
- Calibrated cut-off
- Multiple imputation
Read more about these and other harmonisation techniques in the below articles:

Article
Psychological Distress Across Adulthood: Equating Scales in Three British Birth Cohorts
Take a look at this paper, by Jongsma et al. (2022), for a comparison of these techniques to harmonise psychological distress.
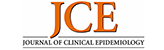
Article
Statistical approaches to harmonize data on cognitive measures in systematic reviews are rarely reported
Read more about different methods for harmonising data in this paper by Griffith et al. (2015).
User guide
Harmonised socio-economic measures
Further information about the coding system used in the harmonisation process for the CLOSER socioeconomic measures dataset can be found in the dataset user guide.
User guide
Harmonised childhood environment and adult wellbeing measures
Further information about the standardisation method used in the harmonisation process for the CLOSER harmonised dataset on childhood environment and adult wellbeing can be found in the dataset user guide.