Measurement invariance
This section briefly introduces the concept of measurement invariance and signposts to a selection of helpful resources.
Checking validity
Testing for measurement invariance is an important statistical step to check the validity of using a particular measure or scale across different groups of people or over different periods of time. If a questionnaire, scale, or other measure shows measurement invariance it means that it is measuring the same construct in the same way across different groups and/or time points. It is important to consider measurement invariance when carrying out cross-study research when the aim is to compare across different studies, countries, cultures, or generations.
Testing for measurement invariance is particularly important for non-standardised scales and latent constructs where we are using a selection of questions or items to measure an underlying unobserved trait, such as the anxiety scale in the example case study.
Confirmatory factor analysis and measurement models
Researchers will most often come across measurement invariance testing in the context of confirmatory factor analysis (CFA) and “measurement models” in the structural equation modelling (SEM) framework, where it is also referred to as factorial invariance. It is also commonly tested under the item response theory (IRT) paradigm by testing differential item functioning.
Types of measurement invariance
There are different types of measurement invariance:
- Configural invariance – where the pattern of factor loadings is the same across groups
- Scalar invariance – where the intercepts or thresholds are equivalent across groups
- Metric invariance – where factor loadings are equivalent across groups
- Residual invariance – where residual variances are equivalent across groups
Example case study: Cross-study research on anxiety
Imagine we are interested in doing some cross-study research on anxiety using data from two British studies, one from 1950 and one from 2010, which both used a selection of questions that make up an anxiety scale.
We want the questions on anxiety in these studies to be measuring anxiety in the same way for the people in 1950 and 2010. If the questions are being interpreted differently between the time points, or somehow measuring anxiety differently due to some other reason, then we can’t be sure that any differences in anxiety we see in our data are due to the factors we are interested in. It could be that the differences we see between 1950 and 2010 are due to the questions and measurement scale that was used, instead of there being an actual difference in anxiety between the generations.
Testing for measurement invariance gives us more confidence that the measurement of the construct we are interested in (in this case, anxiety) is comparable across our groups.
More information and guidance
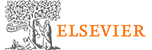
Article
Measurement invariance conventions and reporting: The state of the art and future directions for psychological research
Read more detail about measurement invariance and conventions in reporting in Putnick & Bornstein (2016)
Report
Feasibility of retrospectively harmonising cognitive measures in five British birth cohort studies
See an example of testing for measurement invariance in the CLOSER cross-study data guide on cognitive measures harmonisation feasibility report.